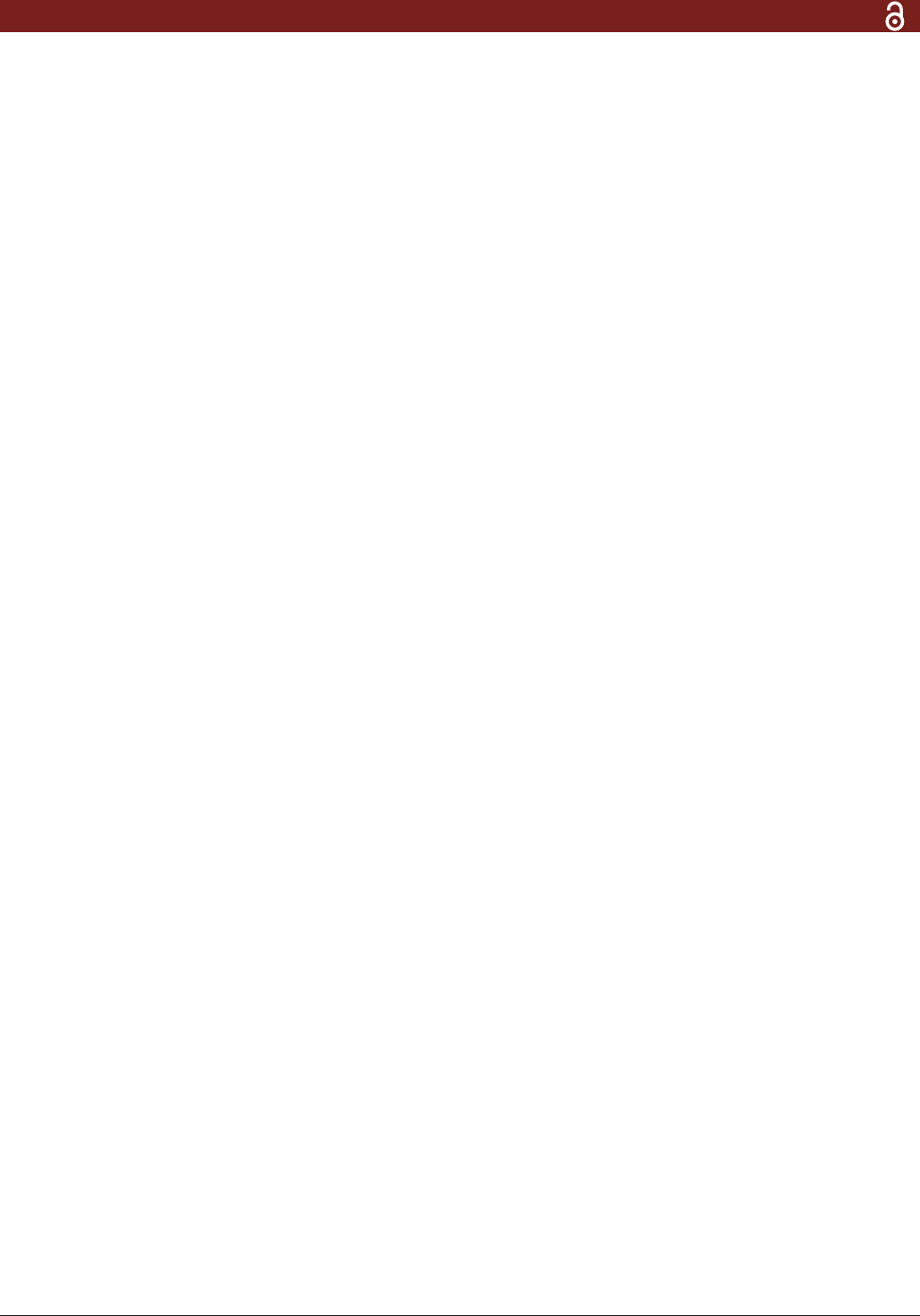
4
SujanM, etal. BMJ Health Care Inform 2022;29:e100516. doi:10.1136/bmjhci-2021-100516
Open access
permits others to distribute, remix, adapt, build upon this work non- commercially,
and license their derivative works on different terms, provided the original work is
properly cited, appropriate credit is given, any changes made indicated, and the use
is non- commercial. See:http://creativecommons.org/licenses/by-nc/4.0/.
ORCID iD
MarkSujan http://orcid.org/0000-0001-6895-946X
REFERENCES
1 Peek N, Sujan M, Scott P. Digital health and care in pandemic times:
impact of COVID- 19. BMJ Health Care Inform 2020;27:e100166.
2 Joshi I, Morley J. Articial intelligence: how to get it right. putting
policy into practice for safe data- driven innovation in health and care.
London: NHSX, 2019.
3 UK AI Council. AI roadmap. London, 2021.
4 Muehlematter UJ, Daniore P, Vokinger KN. Approval of articial
intelligence and machine learning- based medical devices in the USA
and Europe (2015–20): a comparative analysis. Lancet Digit Health
2021;3:e195–203.
5 McKinney SM, Sieniek M, Godbole V, etal. International
evaluation of an AI system for breast cancer screening. Nature
2020;577:89–94.
6 Blomberg SN, Folke F, Ersbøll AK, etal. Machine learning as a
supportive tool to recognize cardiac arrest in emergency calls.
Resuscitation 2019;138:322–9.
7 Komorowski M, Celi LA, Badawi O, etal. The articial intelligence
clinician learns optimal treatment strategies for sepsis in intensive
care. Nat Med 2018;24:1716–20.
8 Fitzpatrick KK, Darcy A, Vierhile M. Delivering cognitive behavior
therapy to young adults with symptoms of depression and
anxiety using a fully automated Conversational agent (Woebot): a
randomized controlled trial. JMIR Ment Health 2017;4:e19.
9 Nagendran M, Chen Y, Lovejoy CA, etal. Articial intelligence versus
clinicians: systematic review of design, reporting Standards, and
claims of deep learning studies. BMJ 2020;368:m689.
10 Sujan M, Furniss D, Grundy K, etal. Human factors challenges for
the safe use of articial intelligence in patient care. BMJ Health Care
Inform 2019;26:e100081.
11 Scott I, Carter S, Coiera E. Clinician checklist for assessing suitability
of machine learning applications in healthcare. BMJ Health Care
Inform 2021;28:e100251.
12 Greaves F, Joshi I, Campbell M, etal. What is an appropriate
level of evidence for a digital health intervention? The Lancet
2018;392:2665–7.
13 Vasey B, Clifton DA, Collins GS, etal. DECIDE- AI: new reporting
guidelines to bridge the development- to- implementation gap in
clinical articial intelligence. Nat Med 2021;27:186–7.
14 Rivera SC, Liu X, Chan A- W, etal. Guidelines for clinical trial
protocols for interventions involving articial intelligence: the SPIRIT-
AI extension. BMJ 2020;370:m3210.
15 Sujan M, Baber C, Salmon P, etal. Human factors and ergonomics
in healthcare AI. Wootton Waven: Chartered Institute of Ergonomics
and Human Factors, 2021.
16 Carayon P, Schoofs Hundt A, Karsh B- T, etal. Work system
design for patient safety: the SEIPS model. Qual Saf Health Care
2006;15:i50–8.
17 Carayon P, Wooldridge A, Hoonakker P, etal. SEIPS 3.0: Human-
centered design of the patient journey for patient safety. Appl Ergon
2020;84:103033.
18 Vicente KJ. Cognitive work analysis: toward safe, productive, and
healthy computer- based work. CRC Press, 1999.
19 Hollnagel E. Why is work- as- imagined different from work- as-
done? In: Wears R, Hollnagel E, Braithwaite J, eds. The resilience of
everyday clinical work. Farnham: Ashgate, 2015.
20 Parasuraman R, Riley V. Humans and automation: use, misuse,
disuse, abuse. Hum Factors 1997;39:230–53.
21 Sarter NB, Woods DD, Billings CE. Automation surprises. In:
Salvendy G, ed. Handbook of Human Factors & Ergonomics. Wiley,
1997: 1926–43.
22 Bainbridge L. Ironies of automation. Automatica 1983;19:775–9.
23 Ruskin KJ, Hueske- Kraus D. Alarm fatigue: impacts on patient safety.
Curr Opin Anaesthesiol 2015;28:685–90.
24 Weld DS, Bansal G. The challenge of crafting intelligible intelligence.
Commun ACM 2019;62:70–9.
25 Levinson DR. OEI- 06- 09- 00091 Hospital incident reporting systems
do not capture most patient harm. Washington, DC: Department of
Health and Human Services, 2012.
26 Saenz MJ, Revilla E, Simón C. Designing AI systems with Human-
Machine teams. MIT Sloan Management Review 2020;61:1–5.
27 Sujan MA, Chessum P, Rudd M, etal. Managing competing
organizational priorities in clinical handover across organizational
boundaries. J Health Serv Res Policy 2015;20:17–25.
28 Sujan MA, Chessum P, Rudd M, etal. Emergency care handover
(ECHO study) across care boundaries: the need for joint decision
making and consideration of psychosocial history. Emerg Med J
2015;32:112–8.
29 Hill RG, Sears LM, Melanson SW. 4000 clicks: a productivity analysis
of electronic medical records in a community hospital ED. Am J
Emerg Med 2013;31:1591–4.
30 Challen R, Denny J, Pitt M, etal. Articial intelligence, bias and
clinical safety. BMJ Qual Saf 2019;28:231–7.
31 Wawira Gichoya J, McCoy LG, Celi LA, etal. Equity in essence: a call
for operationalising fairness in machine learning for healthcare. BMJ
Health Care Inform 2021;28:e100289.
32 High- Level Expert Group On Articial Intelligence. Ethics guidelines
for trustworthy AI. Brussels: European Commission, 2019.
33 Sujan M, Pickup L, Bowie P, etal. The contribution of human factors
and ergonomics to the design and delivery of safe future healthcare.
Future Healthcare Journal 2021;8:e574–9.
copyright.
on August 2, 2024 by guest. Protected byhttp://informatics.bmj.com/BMJ Health Care Inform: first published as 10.1136/bmjhci-2021-100516 on 4 February 2022. Downloaded from